Modelling Child Survival at Birth Data Using Logistic Regression Model: A Case Study of Yobe State Specialist Hospital Damaturu, Nigeria
DOI:
https://doi.org/10.54117/gjpas.v2i2.73Keywords:
Child survival;, Child at birth;, Damaturu;, Modelling;, Logistic regression;Abstract
This research examines factors influencing the survival of child at birth in Yobe State Specialist Hospital using a well-known logistics regression model. A total of 150 data points were collected through transcription from the maternity record of the Hospital to test significant factors that affect survival of child at birth. Analysis of logistic regression model was applied to the data using the Generalized Linear Model (GLM) package in R programming software. The results indicate that Type of delivery and weight at birth have the most significant influence on the probability of child survival at birth at 0.001 level of significance. Correlation analysis results show that all the five variables (age, parity, apgar, birth weight, and type of delivery) have a weak relationship, which implies that there is no multicollinearity in the data. Therefore, these results may help policy makers and health personnel to educate pregnant women on the effect of overweight baby at birth to reduce the incidence of deaths at birth. This study recommend that pregnant women should be educated about the effect of baby weight in a worm as that may increases the chance of caesarean section which in turn may affect the likelihood of child survival at birth. Further studies are suggested to consider factors like educational level, income of level of the family, antennal status, and blood pressure. A more advance community-based survey is recommended since not pregnant women attain formal health care facilities for antennal and postnatal services, which may expose more factors that influence child survival at birth.
References
Esan, D. T., Muhammad, F., Ogunkorode, A., Obialor, B., & Ramos, C. (2022). Traditional beliefs in the management and prevention of neonatal jaundice in Ado-Ekiti, Nigeria. Enfermería Clínica (English Edition), 32, S73-S76.
Emmanuel, A., Kain, V. J., & Forster, E. (2021). Improving the quality of neonatal care in Nigeria through the education of maternity health workers. Journal of Neonatal Nursing, 27(3), 211-215.
Islam, M. A., & Biswas, B. (2021). Socio-economic factors associated with increased neonatal mortality: a mixed-method study of Bangladesh and 20 other developing countries based on demographic and health survey data. Clinical Epidemiology and Global Health, 11, 100801.
Anandalakshmy, P. N., Talwar, P. P., Buckshee, K., & Hingorani, V. (1993). Demographic, socioeconomic and medical factors affecting maternal mortality-an Indian experience. Journal of Family Welfare, 39(3), 1-4.
Adetola, A. O., Tongo, O. O., Orimadegun, A. E., & Osinusi, K. (2011). Neonatal mortality in an urban population in Ibadan, toNigeria. Pediatrics & Neonatology, 52(5), 243-250.
Wilson, J. R., Lorenz, K. A., Wilson, J. R., & Lorenz, K. A. (2015). Standard binary logistic regression model. Modeling binary correlated responses using SAS, SPSS and R, 25-54.
Boateng, E. Y., & Abaye, D. A. (2019). A review of the logistic regression model with emphasis on medical research. Journal of data analysis and information processing, 7(4), 190-207.
Sperandei, S. (2014). Understanding logistic regression analysis. Biochemia medica, 24(1), 12-18.
Scott, D. W. (1990). The New S Language.
Maitra, P., & Pal, S. (2008). Birth spacing, fertility selection and child survival: Analysis using a correlated hazard model. Journal of health economics, 27(3), 690-705.
Audu, L. I., Otuneye, A. T., Mairami, A. B., Mukhtar-Yola, M., & Mshelia, L. J. (2021). Determination of neonatal case-specific fatality rates in a tertiary health institution in North Central Nigeria. BMC pediatrics, 21(1), 1-8.
Akinyemi, J. O., Bamgboye, E. A., & Ayeni, O. (2015). Trends in neonatal mortality in Nigeria and effects of bio-demographic and maternal characteristics. BMC pediatrics, 15(1), 1-12.
Adetola, A. O., Tongo, O. O., Orimadegun, A. E., & Osinusi, K. (2011). Neonatal mortality in an urban population in Ibadan, Nigeria. Pediatrics & Neonatology, 52(5), 243-250.
Mesike, C. G., & Mojekwu, J. N. (2012). Environmental determinants of child mortality in Nigeria. Journal of Sustainable Development, 5(1), 65.
Boerma, J. T., & Bicego, G. T. (1992). Preceding birth intervals and child survival: searching for pathways of influence. Studies in family planning, 23(4), 243-256.
Ronsmans, C. (1996). Birth spacing and child survival in rural Senegal. International journal of epidemiology, 25(5), 989-997.
Madaki, U. Y., Shu’aibu, A., & Umar, M. I. (2023). Mathematical model for the dynamics of bacterial meningitis (Meningococcal meningitis): a case study of Yobe State Specialist Hospital, Damaturu, Nigeria. Gadau Journal of Pure and Allied Sciences, 2(2), 113–129.
https://doi.org/10.54117/gjpas.v2i2.19
Madaki, U. Y., Adamu, U. C., Muhammad, A. A., & Baba, I. A. (2023). Forecasting Infant Mortality Rate using Exponential Smoothing and Moving Averages Techniques. Gadau Journal of Pure and Allied Sciences, 2(1), 40–49. https://doi.org/10.54117/gjpas.v2i1.15
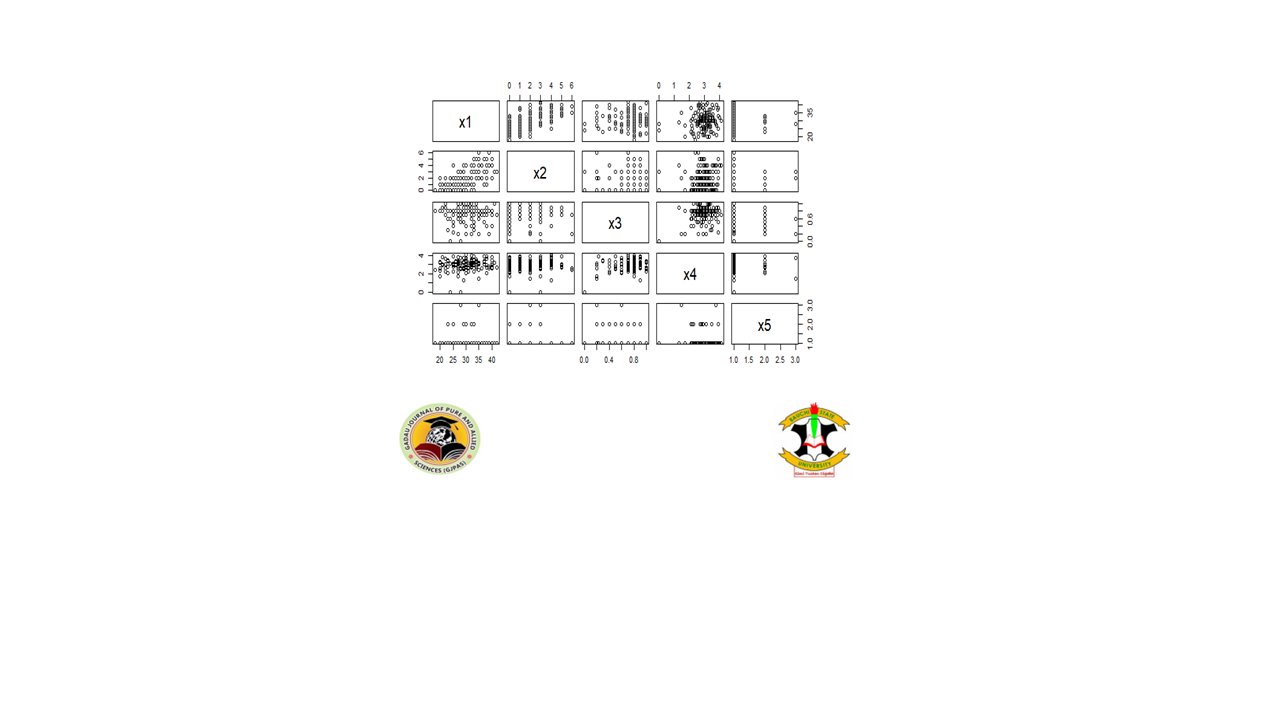
Downloads
Published
How to Cite
Issue
Section
License
Copyright (c) 2023 Gadau Journal of Pure and Allied Sciences

This work is licensed under a Creative Commons Attribution 4.0 International License.